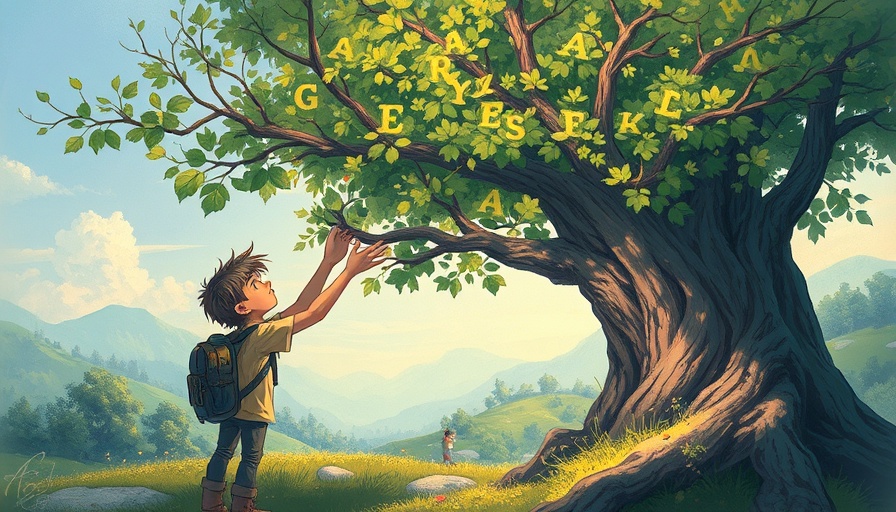
The Rise of Small Language Models in Technology
In the ever-evolving landscape of artificial intelligence (AI), a significant trend is emerging: the shift from large language models (LLMs) to small language models (SLMs). While LLMs, like OpenAI's ChatGPT, sport hundreds of billions of parameters, their smaller counterparts harness a reductionist approach, deploying just a few billion parameters instead. This not only simplifies the computational process but also addresses growing concerns about energy consumption and environmental sustainability.
Why Are Small Models Gaining Popularity?
The appeal lies in their efficiency: SLMs typically require less computational power, making them more accessible for personal devices. As noted by computer scientist Zico Kolter from Carnegie Mellon University, small models can effectively respond to numerous tasks—ranging from health care support as chatbots to data processing in smart devices. This efficiency enables them to be run on laptops or smartphones, shifting AI from powerful data centers into the hands of average users.
Efficiency vs. Power: The Model Dilemma
Though LLMs showcase remarkable capabilities across diverse tasks due to their vast size, the rising costs associated with their training and operation create significant barriers. Google reportedly spent an astonishing $191 million on its Gemini 1.0 Ultra model, which raises questions about the sustainability of such investments. A less energy-intensive solution is the use of SLMs that, as mentioned, excel in specific areas rather than generalizations. For instance, one key benefit of SLMs is the application of knowledge distillation, where large models teach small models using high-quality training data, leading to surprising effectiveness despite fewer parameters.
Innovative Training Techniques: Knowledge Distillation and Pruning
Knowledge distillation, a fundamental technique in training SLMs, streamlines the learning process. Instead of relying on raw, messy data from the internet, large models generate clean, high-quality datasets that optimize the training of smaller versions. This adaptation benefits environments lacking vast computational resources and provides practical solutions for businesses and individuals alike.
Moreover, pruning has become an essential aspect of creating SLMs. By cutting out unnecessary neural connections—similar to how the human brain optimizes itself—engineers make these models more efficient. This innovative approach not only enhances performance but also emphasizes that sometimes, less truly is more in the tech industry.
Looking Ahead: The Future of Language Models
The future of language models seems to hinge on a blend of size and efficiency. Industry experts predict that as AI progresses, SLMs will become increasingly prevalent, particularly for applications that involve specific tasks rather than general use. Environmental concerns, alongside the demand for streamlined tech solutions, will likely spur further innovations in small model capabilities.
In this journey of development, the conversation shifts from sheer power to pragmatic effectiveness. As businesses and developers explore the possibilities of SLMs, we may uncover new applications that enhance everyday interactions with technology, while also aligning with sustainability goals.
Preparing for Change: What You Should Know
For businesses looking to integrate AI technology, understanding the distinctions between SLMs and LLMs will be crucial. The right choice often depends on the intended application and the available resources. As many organizations seek to deploy AI tools, they will need to consider not only performance but also sustainability.
Ultimately, this trend toward small language models signals an exciting shift in the AI paradigm—one characterized by adaptability, efficiency, and broader accessibility. Users from various sectors should prepare to embrace the possibilities presented by these innovative tools.
Write A Comment