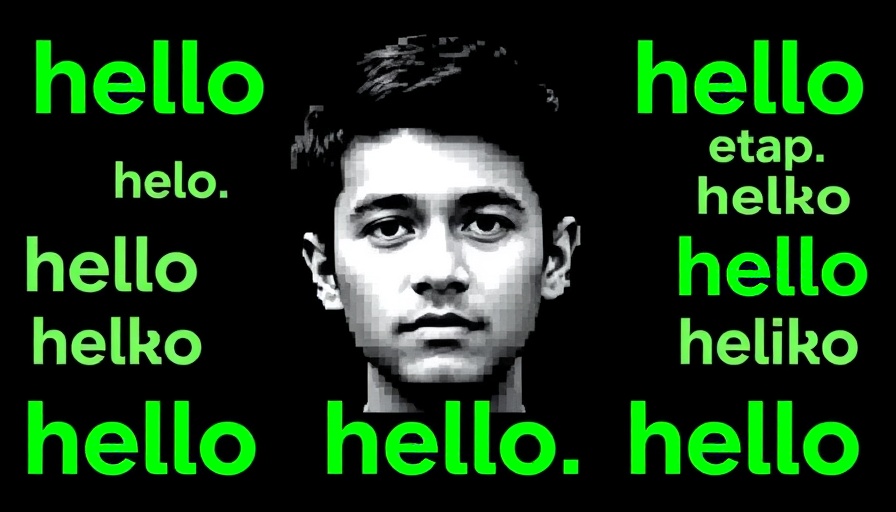
AI Has a Stereotype Problem: Understanding Bias Across Languages
Artificial Intelligence (AI) is often heralded as a leap forward in technological advancement, but researchers are unveiling a troubling side: the spread of outdated stereotypes. A recent conversation with Margaret Mitchell, an authority on AI ethics and leader at Hugging Face, reveals the pressing challenge of AI bias—particularly how it persists across languages and cultures.
Shifting Perspectives: The SHADES Dataset and Its Importance
Mitchell’s team created the SHADES dataset aimed at evaluating bias in AI models beyond just the English language. This innovative approach, borne out of the collaborative BigScience project, encourages understanding and analysis through rigorous testing of different identity characteristics—much like the Gender Shades project, which focused on gender and skin tone. Unlike existing multilingual bias resources that often rely on machine translations, SHADES emphasizes human translations, capturing subtleties inherent in various cultures.
Why Broaden the Focus Beyond English?
Focusing exclusively on English-speaking contexts may significantly narrow the understanding of AI biases. In many cases, the complexities and nuances of cultural identity can dramatically shift the narrative in non-English languages, making a broader lens essential for a true understanding of AI's impact. As we navigate through a more globally connected world, recognizing these biases in local contexts is vital to ensuring fairness in AI development and its applications.
The Broader Impact of AI Bias on Society
The influence of AI extends far beyond the tech industry and into daily life, affecting everything from hiring practices to law enforcement. As AI systems often mirror the biases present in their training data, the repercussions can be particularly damaging to marginalized communities. Understanding and addressing these biases can contribute to a fairer society, paving the way for technologies that reflect our diverse world accurately.
Future Predictions: How Can We Combat AI Bias?
Moving forward, it is crucial to implement strategies that consider the cultural and linguistic differences at play in AI systems. The SHADES dataset represents only the tip of the iceberg; future developments should prioritize inclusivity in AI through diverse datasets, inclusive training methodologies, and a multi-disciplinary approach to AI ethics. By doing so, developers can create systems that serve a wider audience while minimizing harm.
Diverse Perspectives: Counterarguments and Solutions
Some might argue that focusing on bias can hinder AI progress or that attempting to mitigate bias increases complexity in development. However, ignoring these biases can lead to systems that reinforce stereotypes and inequality, ultimately stagnating innovation. Rather than creating barriers, the emphasis on ethical AI development can lead to broader acceptance, wider engagement, and more innovative solutions.
Empowering Future Generations of AI Developers
It's not just about correcting biases; we must also empower the next generation of AI developers and researchers. Educational programs that emphasize ethics in AI from the beginning can cultivate a critical perspective within future technologists, ensuring they prioritize these values as they advance the field.
A Call for Action: Engaging in Ethical AI Development
The conversation around AI bias is just beginning. As consumers, developers, and researchers, everyone has a role in promoting ethical AI practices. By advocating for inclusive training data and transparency in AI models, we can collectively push toward a future where technology reflects the diversity of the world it serves. Start by engaging in discussions around these issues, both online and in your communities.
Write A Comment